What LDT can do for you
What LDT can do for you
This page lists a few examples of how LD scores can be used in practice to do error analysis, explain downstream task performance, and also guide model development and optimization.
Contents
Explaining performance on downstream tasks
Question: why is model X beating model Y on task Z?
Answer: model X is doing something differently from model Y. Let us consider the example of CBOW, GloVe and Skip-Gram models with select LD scores and task performance results.
CBOW | GloVe | SG | |
---|---|---|---|
LD factors | |||
SharedMorphForm | 51.819 | 52.061 | 52.9 |
SharedPOS | 30.061 | 35.507 | 31.706 |
SharedDerivation | 4.468 | 3.938 | 5.084 |
Synonyms | 0.413 | 0.443 | 0.447 |
Antonyms | 0.128 | 0.133 | 0.144 |
Hyponyms | 0.035 | 0.035 | 0.038 |
OtherRelations | 0.013 | 0.013 | 0.013 |
Misspellings | 13.546 | 9.914 | 12.809 |
ForeignWords | 2.147 | 1.976 | 1.793 |
ProperNouns | 30.442 | 27.278 | 27.864 |
Numbers | 4.313 | 3.147 | 3.64 |
LowFreqNeighbors | 94.778 | 66.51 | 96.109 |
HighFreqNeighbors | 3.421 | 15.697 | 2.513 |
NonCooccurring | 88.97 | 67.904 | 90.252 |
CloseNeighbors | 3.102 | 0.16 | 2.278 |
FarNeighbors | 25.209 | 49.934 | 21.41 |
Downstream tasks | |||
POS-tagging | 87.660 | 83.800 | 87.860 |
Chunking | 77.530 | 66.100 | 78.230 |
NER | 75.210 | 69.620 | 75.720 |
Relation class. | 74.780 | 71.050 | 74.800 |
Subjectivity class. | 89.800 | 89.160 | 89.920 |
Polarity (sent.) | 75.900 | 74.600 | 76.860 |
Sentiment (text) | 82.220 | 82.240 | 82.730 |
SNLI | 69.290 | 69.510 | 69.740 |
Skip-Gram consistently outperforms both CBOW and GloVe, but the patterns are different:
- LD scores for CBOW and Skip-Gram are mostly similar, but CBOW is always slightly trailing behind on meaningful relations such as synonyms, antonyms, and morphological relations, while being ahead on noise. It is also slightly trailing behind on all downstream tasks.
- GloVe appears to be quite different from both CBOW and Skip-Gram in the distributional factors: in particular, it is much worse at finding neighbor words if they are low-frequency or not cooccurring with the target word in the corpus. Thus it looks that it is less efficient at deducing semantic information without direct distributional evidence.
Parameter search
Question: how do I maximize amount of information type X?
Answer: explore the impact of model hyperparameters on that information type.
Example: In use case 1, GloVe model behaved rather differently from Word2Vec in terms of distributional information. In particuar, it was much worse at capturing relations between words that did not co-occur in corpus, but had to be inferred on the basis of larger semantic patterns. Let us see how this factor varies with different vector size of the embedding.
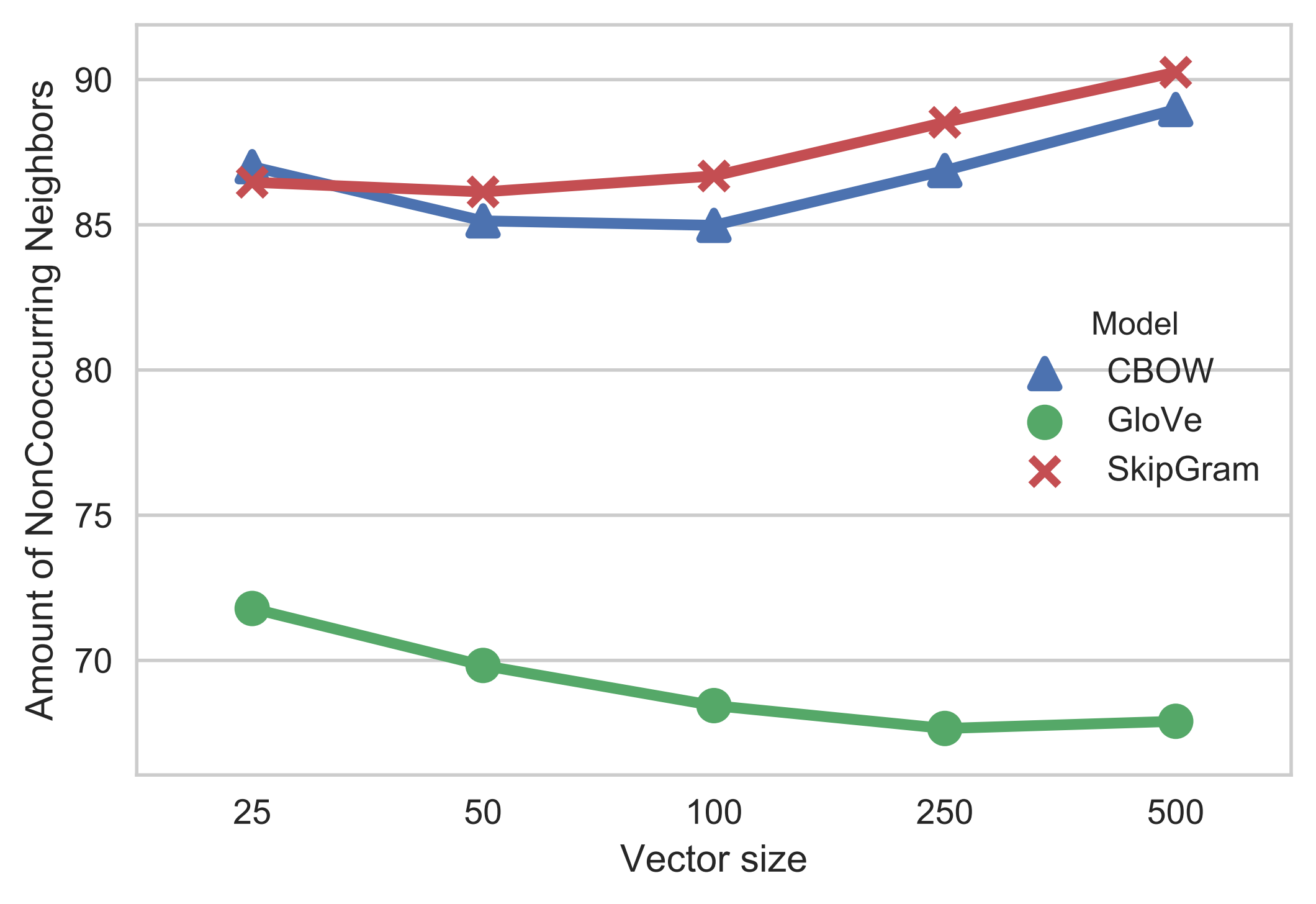
Effect of vector size on inference of relations between non-cooccurring words
Surprisingly, GloVe does the best at this at the lowest dimensionality, perhaps due to the fact that lower dimensionality is forcing it to look for wider patterns. But even that it is nowhere close to Word2Vec scores.
Hypothesis testing in model development
Question: My model should be specializing in X, how do I make sure?
Answer: Profile your models for X.
Example: Dependency-based embeddings bring together words that can perform similar syntactic functions, as opposed to standard linear bag-of-words. This could mean that dependency-based embeddings have a higher ratio of synonyms in word vector neighborhoods.
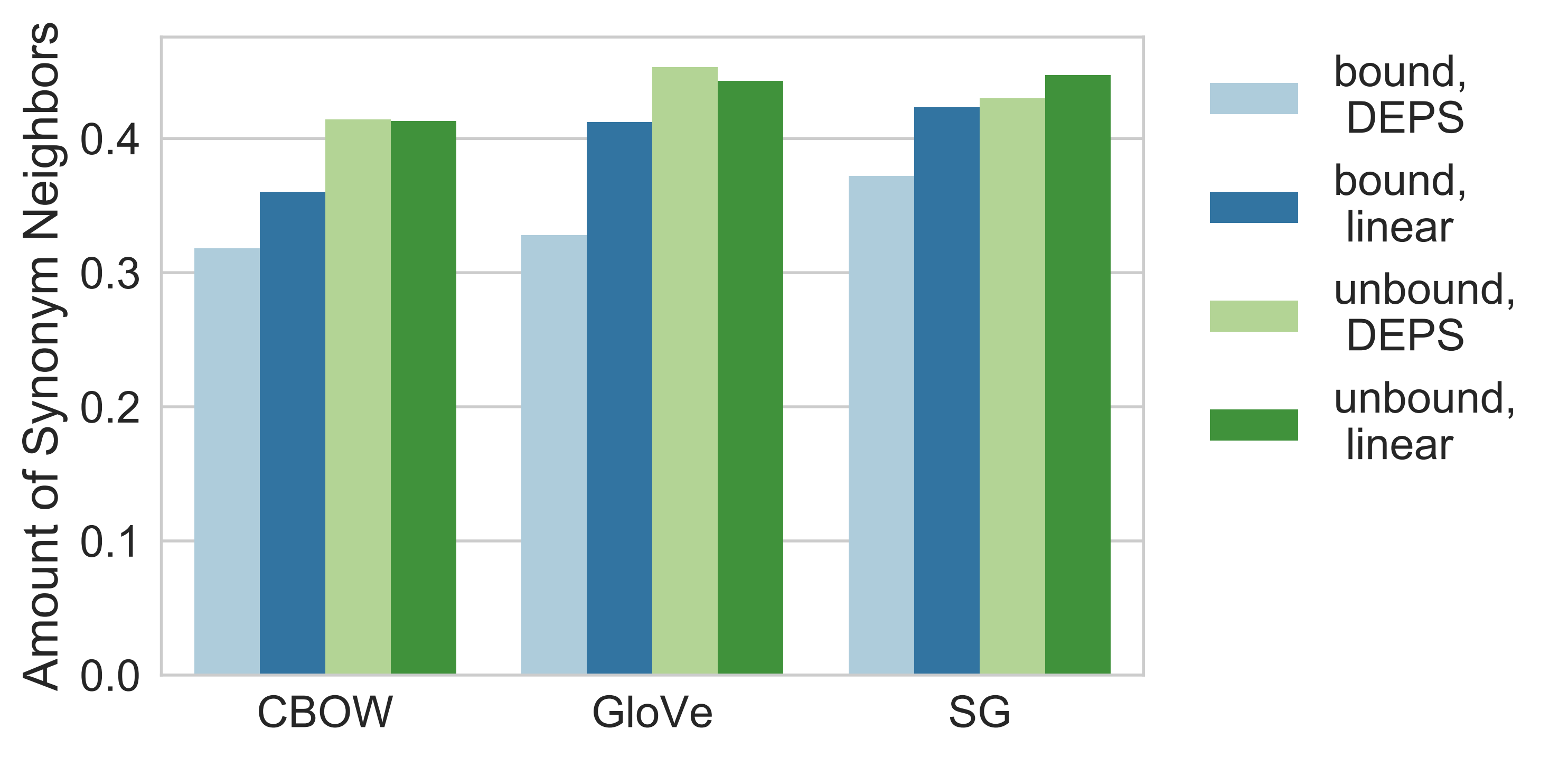
Effect of context type on specialization in synonymy
It appears that dependency-based embeddings actually do not outperform regular bag-of-words models.